RLHF|DPO, GRPO
本文梳理了 DPO,GRPO 的主要特点、亮点以及相关资源链接。
DPO
先来回顾以下 PPO,采用 PPO 的 RLHF 会经过 reward model tuning 和 Reinforcement Learning 2 个步骤:
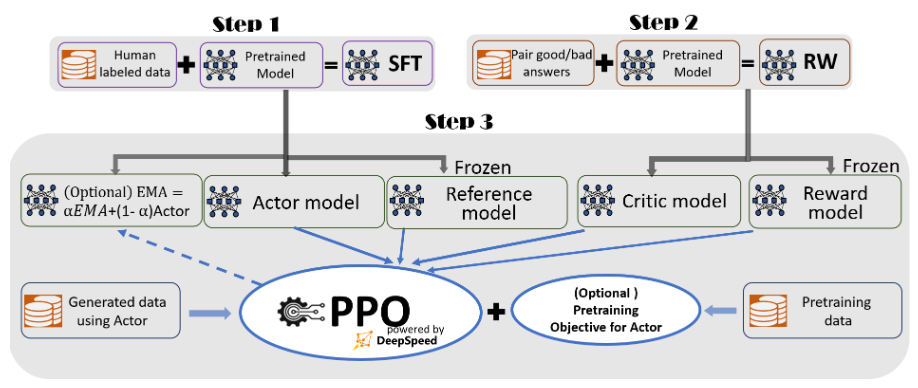
首先在上图的 Step 2 中训练 reward model ,优化正负样本之间的距离
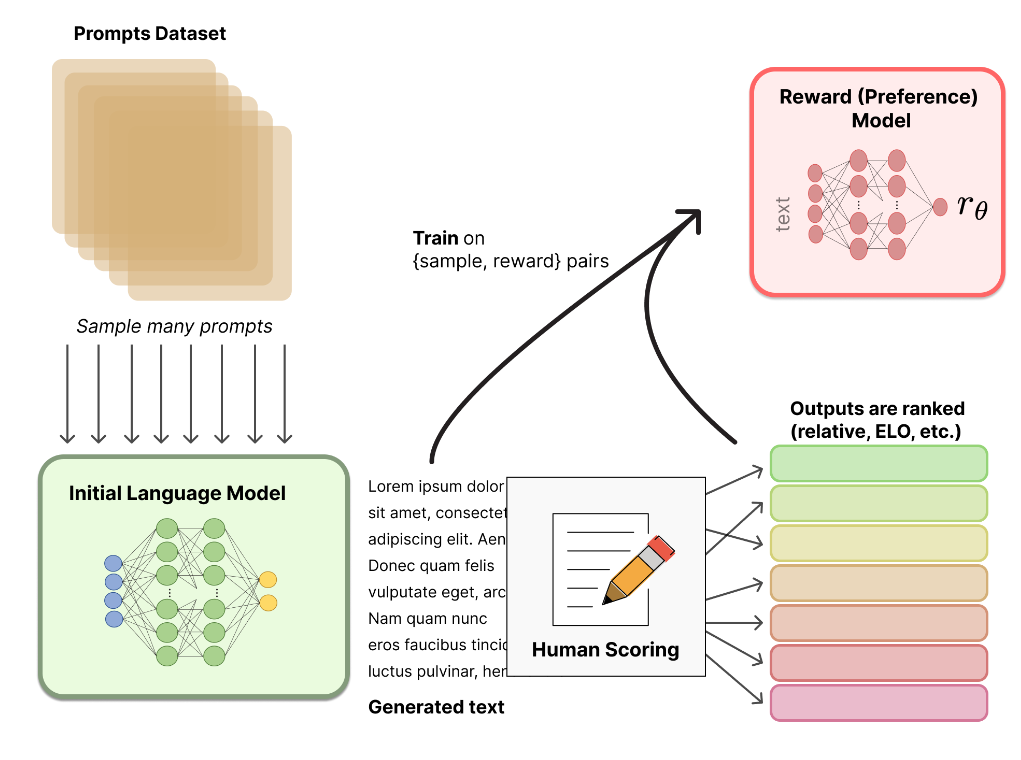
而后在 Step 3 中采用梯度上升优化 LLM,目标函数为:
大致流程如下:
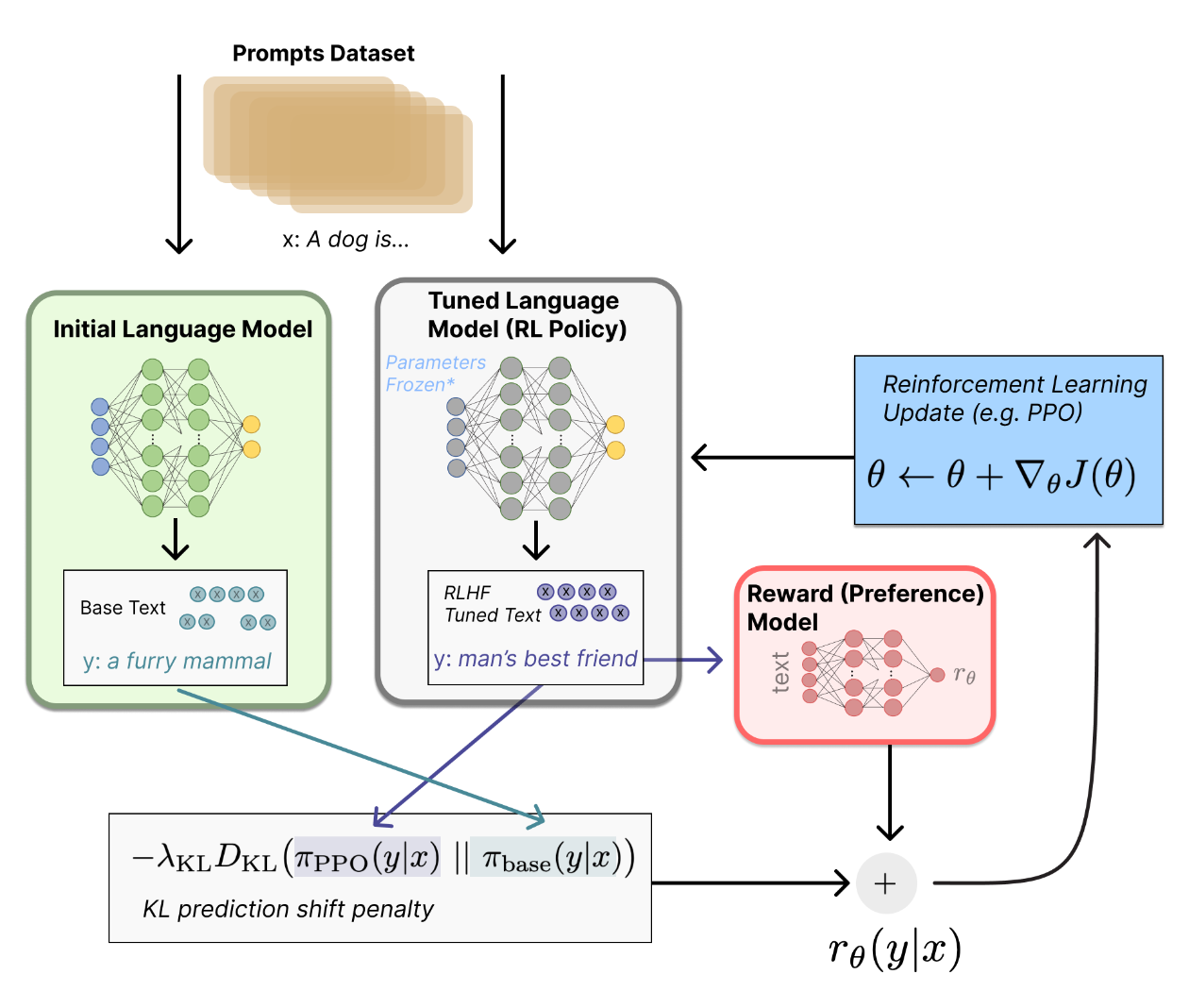
更多 PPO 细节可以参考 RLHF 基础笔记。
DPO 的重点在于:将 PPO 的目标函数转化为了简单的 binary corss-entropy 目标函数。
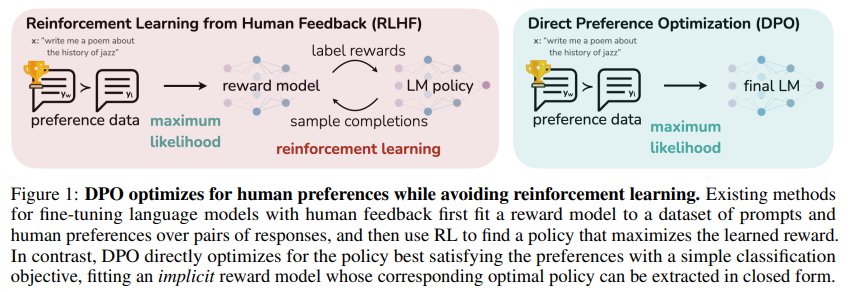
其中 为 partition function:
我们可以定义:
于是公式 可以整理成:
可以看到,上式中, 是不受 能影响的。因此当 KL 散度为 0 时,以上式子有最优解,即:
但以上式子中, 比较难求,因此我们对上式进行以下变换,可以得到:
将以上式子带入,reward function 的损失函数中,得到:
因此,优化时候采用的数据集格式与训练 reward model 相似,也是 的格式,在优化 时,不计算 reward model,也可以实现 RLHF 的效果。
参考 trl
的 dpo loss 计算方式,大致流程为:
def dpo_loss(
self,
policy_chosen_logps: torch.FloatTensor,
policy_rejected_logps: torch.FloatTensor,
reference_chosen_logps: torch.FloatTensor,
reference_rejected_logps: torch.FloatTensor,
) -> Tuple[torch.FloatTensor, torch.FloatTensor, torch.FloatTensor]:
# ... 省略部分代码
logits = (policy_chosen_logps - reference_chosen_logps) - (policy_rejected_logps - reference_rejected_logps)
if self.loss_type == "sigmoid":
losses = (
-F.logsigmoid(self.beta * logits) * (1 - self.label_smoothing)
- F.logsigmoid(-self.beta * logits) * self.label_smoothing
)
# ... 省略其他 loss_type
return losses, # ... 省略其他输出
在优化时候,可以与 sft loss 一起优化,当同时优化 SFT + DPO 时,sft loss 只计算 chosen 样本对应的 loss。
GRPO
论文:DeepSeekMath: Pushing the Limits of Mathematical Reasoning in Open Language Models
GRPO 在 DeepSeek V2 中采用了,GRPO 在训练过程中,不需要 Value Model,因此也能够减少 RL 训练过程中的资源消耗。
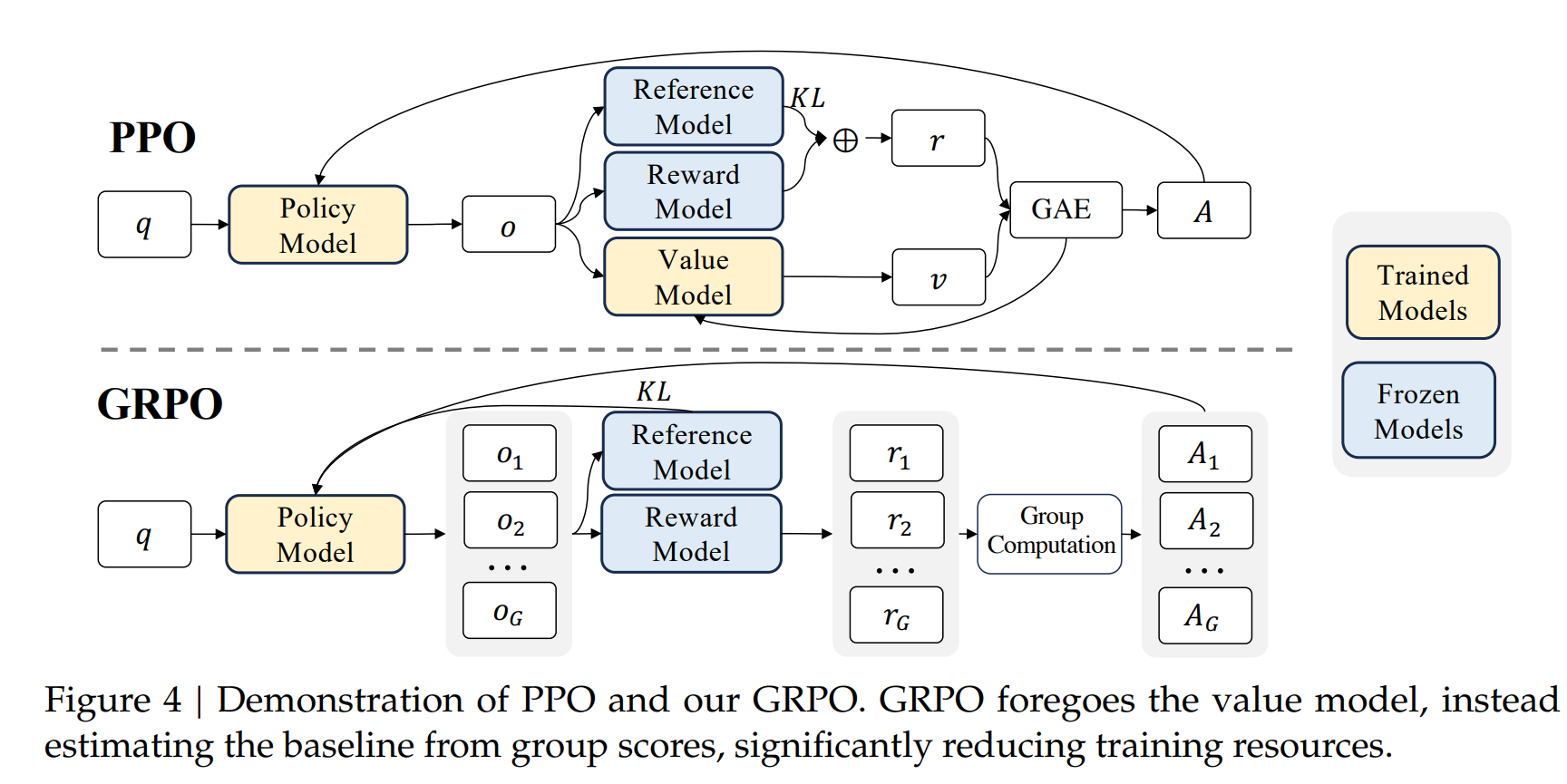
GRPO 的目标函数为:
GRPO 的步骤大致为:
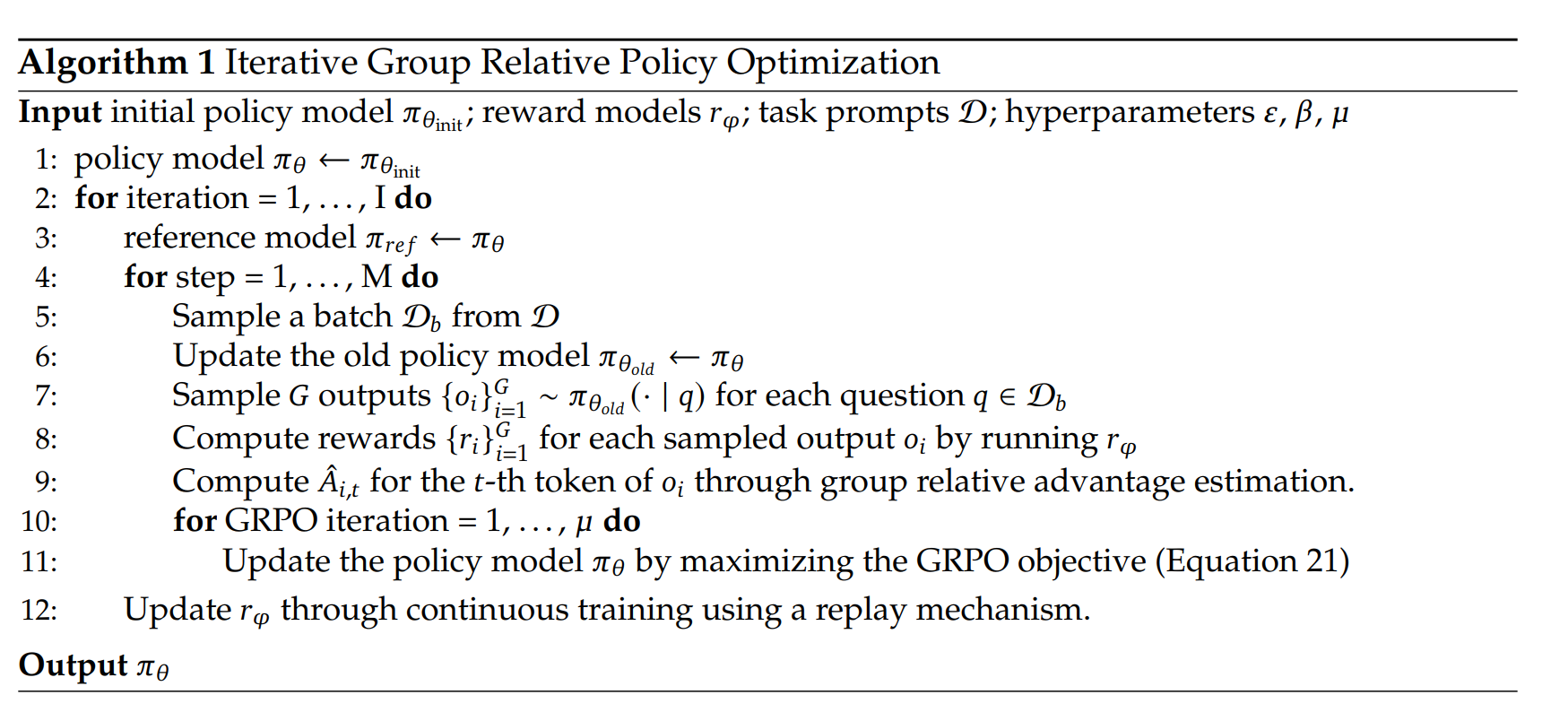
其中,advantage 。
参考
RLHF 相关训练代码示例:
https://github.com/huggingface/alignment-handbook
https://github.com/OpenRLHF/OpenRLHF
https://github.com/hiyouga/LLaMA-Factory